The growing complexities and innovations in Business Process Mining
Written by Alan Del Piccolo and Sylvio Barbon Junior
19 February 2025 · 8 min read
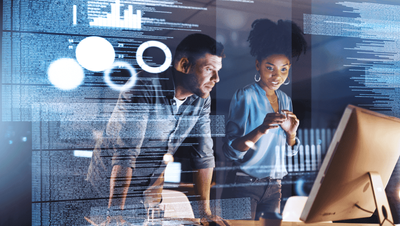
In an earlier blog post we introduced the concept of Process Mining, with a snapshot of its current state of maturity and its possible paths. At the end of such a contribution we provided a glimpse of how Cardanit fits into the picture.
It‘s time to pick up where we left off to account for the latest developments of our platform, and to cast our look further beyond thanks to the contribution of an expert in Process Mining, Professor Sylvio Barbon Junior of the University of Trieste.
Cardanit’s path from being a process and decision editor towards being a comprehensive, all-around analysis tool has taken big steps forward thanks to the continuous upgrade of the simulation capabilities of the platform. While on the one side the list of parameters provided by Cardanit to customize process simulation is steadily growing, on the other the support for the analysis of the results of a simulation is ever-increasing. Tables and heat-maps can be created and customized to gather insights on what is actually happening inside our processes.
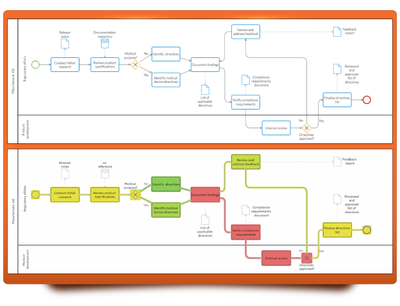
To customize a simulation is essential to tailor a customer’s “what-if” scenarios. In short, the classes of simulation parameters that Cardanit offers so far are: resource management, trigger parameters, cost tracking for tasks, and time parameters and calendars (keep an eye on our Release notes to stay updated).
But where do we get these parameters from in the first place? Where do we start? Such pieces of information can be seen as a component of the “as-is” picture of our processes. Ideally, an initial setup of the simulation parameters, along with a correctly modeled process, should produce simulation results that are comparable to those of the actual process we started from.
Process Discovery, the first task of Process Mining, extracts information from the event logs of past executions to describe the “as-is” picture of our process. It’s fitting that such an extraction procedure may be extended to produce baseline values for the simulation parameters, alongside a model of the actual process. The model and the parameters could then be fed into Cardanit’s simulation engine to start a cycle of simulation, analysis and refinement. Or, in terms of Business Process Management Life Cycle, execution, monitoring and optimization.
Of course, extracting such information is complicated. To begin with, process data are often unstructured, they can be made of different parts coming from different sources, and they often contain spurious information, such as outliers. To convert them into a coherent event log, which is the starting point for Process Mining tasks, can be troublesome. Our colleague Alan Del Piccolo, from the ESTECO Research and Development department asked an expert, Professor Sylvio Barbon Junior. Sylvio is Associate Professor at the Department of Engineering and Architecture at the University of Trieste, and is currently part of the Machine Learning Lab.
Integrating unstructured data into Process Mining for enhanced insight
Alan Del Piccolo: Professor, what are the benefits and the pitfalls of dealing with diverse, unstructured sources of data for Process Mining?
Sylvio Barbon Junior: While structured data easily aligns with the quantitative nature of event logs, unstructured data (emails, free-form text notes, images, etc.) encapsulates a vast amount of process-related information that is often overlooked due to its complexity. Integrating this type of data into process mining involves extracting relevant data points from unstructured sources and transforming them into a structured format that can be logged and analyzed. Techniques such as natural language processing (e.g., Large Language Models as ChatGPT), image recognition, and machine learning are used for this transformation, enabling deeper insights and enhancing the accuracy of the process models.
Alan Del Piccolo: Can you describe a real-life scenario for this approach?
Sylvio Barbon Junior: For example, enriching process activity descriptions in healthcare process mining with additional data sources such as medical reports, sensor results, and medical images can substantially enhance predictive capabilities. Typically, healthcare process models might only capture basic attributes like activity type, timestamp, and involved personnel. However, by integrating richer data forms, the model's accuracy and contextual depth increase significantly.
Incorporating textual information from medical reports through natural language processing techniques allows for a deeper understanding of patient conditions and treatment outcomes. Similarly, sensor data provides real-time insights into a patient's physiological state, which can help predict necessary interventions or outcomes. Additionally, medical images such as X-rays or MRIs, when analyzed with advanced image recognition technologies, offer critical visual data that can detect patterns or anomalies associated with specific process outcomes.
However, this approach comes with challenges. These include: managing the complexity and volume of data, ensuring privacy and security compliance given the sensitive nature of patient data, and overcoming technical hurdles related to data integration and interoperability. Despite these challenges, the opportunities for improving predictive accuracy, personalizing patient care, and enhancing operational efficiency are significant. By providing a more detailed and accurate view of patient care processes, enriched process models not only support medical professionals in making better-informed decisions, but also improve overall patient care outcomes.
Adding Dimensions to Process Mining
Alan Del Piccolo: Present-day process modeling and process mining usually deal with a single entity at a time, exploring and manipulating the activities pertaining to such an entity in isolation. This approach is called “Case-centric”. However, it’s shown to lose the information related to the interaction of our entity with the other entities in our process, leading to an incorrect modeling. (Think of modeling a purchase from an online store without taking into account partial returns, split deliveries and so on).
This further motivates the impulse to treat richer, multi-dimensional data in the form of objects.
Sylvio Barbon Junior: The demand for multidimensional data in event logs is escalating as businesses seek to incorporate external variables (like market conditions, customer feedback, and supply chain dynamics) that influence processes. This integration enables a more holistic view of process performance and its influencing factors. However, it also introduces complexity in terms of data variety, volume, velocity, and veracity (the four Vs of big data). Managing this multidimensional data requires advanced data integration techniques and robust data management systems to ensure data quality and accessibility. To address these challenges, efforts are being made to develop Object-Centric Process Mining (OCPM), which supports the structuring of event logs into Object-Centric Event Logs (OCEL). This approach allows different dimensions to be represented as distinct objects, broadening the scope for a wide range of possibilities, including the application of more recent AI methods. By organizing data in this way, OCPM facilitates more comprehensive and flexible analyses, enabling deeper insights into complex, multidimensional process data.
Predicting the Future Right
Alan Del Piccolo: So far we’ve been dealing with post-hoc analyses of processes, which aim to gain understanding of what has happened and why. However, “what will happen?” is another fundamental question of Process Mining. If we could somehow anticipate the result of a computation while the process is still running, then we might correct its course in time to steer it towards more desirable results.
How can Process Mining help us foresee the rest of the execution of our process, and perform the appropriate correcting actions if necessary?
Sylvio Barbon Junior: A key task of Process Mining is Predictive Process Monitoring. Leveraging historical data, predictive monitoring forecasts future states of ongoing cases in a process. It predicts metrics like the next activity, the remaining time of a process, and the outcome of process instances, helping managers and executives make informed decisions.
Predictive Process Monitoring stands out as a forward-looking component of Process Mining. It helps organizations anticipate future process behaviors and outcomes. The challenge here lies in balancing the timing of predictions—too early, and the prediction may lack accuracy; too late, and it may be irrelevant.
Early predictions provide longer reaction times but can be less accurate as they must predict further into the future with less information. Conversely, late predictions tend to be more accurate but offer less time for corrective actions. This balance is crucial for operational efficiency and can significantly impact strategic planning and real-time decision-making.
Optimizing this balance involves enhancing the algorithms with machine learning models that can learn from a multitude of scenarios and adjust their predictions based on real-time data feeds. Furthermore, incorporating more contextual and external data can refine these predictions, making them both timely and relevant.
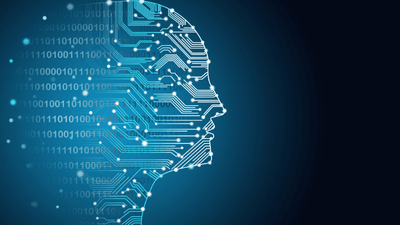
The Impact of AI on Process Mining
Alan Del Piccolo: With the progress in the field of AI that we’re witnessing on a daily basis, do you envision an impact into the current Process Mining tools and practices?
Sylvio Barbon Junior: Tasks such as Process Enhancement, Conformance Checking, and especially Predictive Process Monitoring extensively utilize techniques from Machine Learning (ML) and Deep Learning (DL). These advanced computational methods enable more sophisticated analyses and improvements of process models by identifying patterns and predicting future behaviors more accurately.
Process Mining is evolving from a static analytical tool into a dynamic, predictive, and highly integrative technology capable of handling complex, multidimensional datasets and unstructured information. As businesses operate in increasingly volatile environments, the ability to quickly adapt and optimize processes becomes critical. Process Mining not only provides the visibility needed to understand and improve existing processes but also the predictive capabilities to anticipate and adapt to future challenges. Artificial Intelligence, especially Machine Learning and Deep Learning, plays a crucial role in this mining strategy by enhancing the intelligence of practitioners and engineers dealing with complex process scenarios.
The integration of advanced data processing techniques and AI into process mining will be paramount in overcoming the inherent challenges of data complexity and prediction timing. This evolution will undoubtedly unlock new dimensions of process intelligence, driving efficiency and innovation across various industries.
Unlocking Hidden Opportunities
In conclusion, our discussion highlighted the motivation behind the new developments in the field of Process Mining. Unstructured data integration, object-centric analysis, and AI-supported predictions represent the near future of such a discipline. By leveraging these innovative approaches, organizations can gain unparalleled insights into their operations, leading to enhanced efficiency and informed decision-making. As we continue to navigate complex business environments, embracing Process Mining will be pivotal in driving continuous improvement and maintaining a competitive edge. There is a long development roadmap ahead of Cardanit, but our path will surely cross its way with Process Mining shortly.
Further reading
Process Mining: the real value for Process Management
Process Simulation: from Automation to Digital Twin
The five steps of the Business Process Management lifecycle
Alan Del Piccolo has a PhD in Computer Science from Ca’Foscari University of Venice, with a specialization in Human-Computer Interaction. He started working for ESTECO SpA in 2019 in the Research and Development department; then, he moved to Product Development. After four years as a software engineer he is back to being a researcher.
Alan Del Piccolo has a PhD in Computer Science from Ca’Foscari University of Venice, with a specialization in Human-Computer Interaction. He started working for ESTECO SpA in 2019 in the Research and Development department; then, he moved to Product Development. After four years as a software engineer he is back to being a researcher.
Graduated in Computer Science and Computer Engineering with a master's and PhD in Computational Physics. From 2009 to 2012 Sylvio was Assistant Professor at UEMG (Brazil), and Adjunct Professor at State University of Londrina (UEL) from 2012 to 2021. In 2017 he was Visiting Researcher at Università degli Studi di Milano and developed a post-doctorate research project at Università di Modena e Reggio Emilia. In 2021 started as Associate Professor at University of Trieste, Italy. Sylvio's research interests include Digital Signal Processing, Pattern Recognition, Machine Learning and Computer Vision.
Graduated in Computer Science and Computer Engineering with a master's and PhD in Computational Physics. From 2009 to 2012 Sylvio was Assistant Professor at UEMG (Brazil), and Adjunct Professor at State University of Londrina (UEL) from 2012 to 2021. In 2017 he was Visiting Researcher at Università degli Studi di Milano and developed a post-doctorate research project at Università di Modena e Reggio Emilia. In 2021 started as Associate Professor at University of Trieste, Italy. Sylvio's research interests include Digital Signal Processing, Pattern Recognition, Machine Learning and Computer Vision.
A business is only as efficient as its processes. What are you waiting to improve yours?