Process Mining: from expectations to the real value for Process Management
Written by Alessandro Turco
28 May 2024 · 7 min read
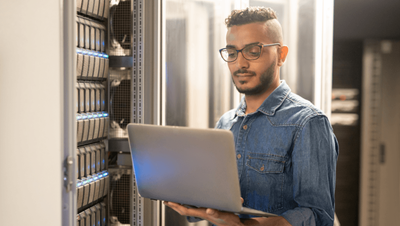
Who am I to question Gartner’s opinion?
In August 2023 the Gartner’s report “Hype Cycle for Revenue and Sales Technology” said that Process Mining is still on the innovation trigger stage and it is expected to reach the plateau of productivity in 2-5 years after passing through all other phases of the Hype Cycle model. My personal and humble opinion is that, if we separate “standard” process mining from its generative AI enhanced variants, the former can be placed on the slope of enlightenment as a well established technology.
What is Process Mining?
Process Mining is a set of techniques which lies in the intersection between Process Science and Data Analysis. The leading idea is to leverage data in order to better manage processes.
For example, imagine a company with unstructured processes that uses a CRM software to record interactions with customers. With Process Mining they can analyze the log of the information system and identify the most common paths in the customer journey as well as the most painful deviations from them.
Process Mining algorithms require a well structured log to start with. The richer the log, the deeper the analysis. However, the essential pieces of information are only three:
- a timestamp (when the activity/event happened)
- a name for the activity/event (a concise and unique label for repetitive entries in the log)
- a case identifier for the process instance (for example the order number that brings together different entries in the log)
With such a log, it is possible to reconstruct (mine) the process model. This step requires a little bit of tuning to choose the right level of abstraction for the process and to select the interesting subset of traces (i.e. the footprints of a process instance in the log) to focus on. All modern Process Mining software have graphical interfaces to facilitate this work.
Beyond Process Mining: conformance checking
Another possible application of Process Mining is the case of a well structured company, with process maps hanging on every wall and printed for every employee. If the activities of such a company are operated through an information system, the logs can be manipulated to assess the compliance with the prescribed policy. This inspection is usually called “conformance checking” in the Process Mining community.
Conformal checking is a necessity in strictly regulated businesses, like banks or insurances, because of laws and regulations. But it can uncover improvement opportunities when the prescribed process is changed: sometimes people in their daily activities take shortcuts which may end up being a natural enhancement to the prescribed process. Process Mining can measure the frequency of these alternative paths, their pros and cons and open the way for process reengineering.
Further possibilities with Process Mining
Those are just a couple of examples. The truth is that once you collect data about your process and analyze them with proper mathematical, statistical tools, you can extract useful information both looking at the past (what we did in a sub-optimal way?) and to the future (considering our history, what is the expected outcome of a process with certain attributes?). Process Mining does this work (Data Analysis) always keeping in mind that the goal is Process Management, a discipline where the improvements sum up with the repetition of process instances and where people are usually the fundamental asset.
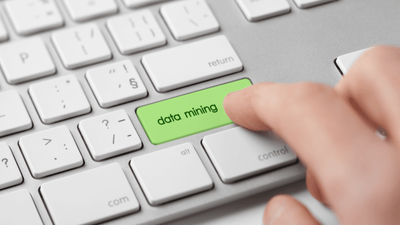
What is the Hype Cycle?
From its privileged and experienced point of observation, Gartner observed a common path in the spreading of new technologies and theorized the “Hype Cycle”. The hype cycle is a line in a cartesian graph with time on the horizontal axis and a measure of the effectiveness and the availability of the technology under examination on the vertical one. Sometimes this measure is the expectation that people have on the technology, sometimes the visibility, but probably the right word is exactly “hype”.
The five steps of the Hype Cycle
Although time might flow at different speed for different technologies and for different stages of the same one, Gartner asserts that any innovation in the digital and computational world passes through five steps:
- Technology trigger, where the technology starts spreading and the expectations rise quickly.
- Peak of expectations, when that innovation seems the solution to any related problem.
- Trough of disillusionment, a quick drop in the hype usually due to the fact that the new technology is not mature enough to meet the huge expectations.
- Slope of enlightenment, a new and more solid increment of the expectation that now, considering the advanced development of the technology, resembles more confidence than hype.
- Plateau of productivity, when the expectations do not grow too much, but you know exactly what the technology can do and how to profit from it.
Why is it a cycle?
I’ve always wondered why this path is called a “cycle”. I think there is a link to the theories of sustaining against disruptive innovation that predict periodic revolutions on the market. This is where incumbent firms/products are disrupted by newcomers whose inevitable path is to become established and being replaced by the next wave. In this sense, after a technology reaches the plateau of productivity, it’s ready to be substituted with a new one starting its path on the Hype Cycle. However, I tend to believe that this latter part is much more true for B2C contexts, considering the longevity of some very productive tools in the B2B arena.
Is Process Mining a mature technology?
We can now go back to the original question: is Process Mining still in the first stage of the Hype Cycle? As I said at the beginning, it’s worth distinguishing between the first wave of Process Mining tools and techniques and everything related to generative AI.
Both Data Science and Process Science fit very well with machine learning, neural networks, natural language processing and their sum, generative AI.
The combination of human expertise and automatic learning, predictive and prescriptive analytics helps handle processes with tangled interactions and with huge databases. These have to be filtered, ordered, sorted, analyzed and only then they become the source of new information. The scientific research and the product market explored different ways to exploit generative AI for Process Mining purposes, that we can wrap up in two main categories: tools that help less-technical people to use advanced techniques (democratization) and tools that allow expert analysts to expand even further their capabilities.
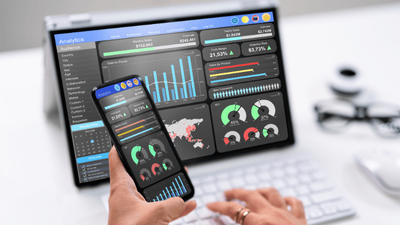
Artificial Intelligence and Process Mining in action
The first category includes all the applications where “coding” is replaced by natural language:
- you can extract a process map from a normative textbook
- you can retrieve KPIs and aggregated statistics by simply asking question
- you can also automatically perform long sequences of data manipulations with a short instruction
Predictive and prescriptive analytics belong to the second category: once the AI is trained, it can start providing possible future scenarios, with associated probabilities, or suggest improvements or corrective actions. Then, of course, it is possible to combine the two and start asking questions in an interactive way: what is the most probable next step in my process instance? What can I do to accelerate a delayed task? How can I conquer the world?
Expectations growing
There is no limit in this direction, or this is the common perception at the moment (if we exclude some post-apocalyptic books and films). Probably, this is an indication that Process Mining is in the technology trigger phase and it has not reached the peak of expectations yet. But this is not due to the intrinsic nature of Process Mining, instead I see a contamination of generative AI which is in the initial expansion phase. If we look at the core elements of Process Mining, there is a solid body of knowledge and of practice that pushes it well ahead in the Hype Cycle in my opinion.
Is there disillusionment in the future?
If we admit that, we must also accept that somewhere in the past Process Mining reached the trough of disillusionment: where was it? The number of scientific papers related to the topic increased exponentially in the last 15-20 years, without any evidence of disillusionment (even during the pandemic). My belief is that the disillusionment was not in the knowledge, nor in the market, but in the practice instead. Every Process Mining initiative in the real world that I am aware of (they can be a limited fraction, I know) passed through a desperation moment: sometimes the initial log was too scattered, sometimes it was unclear the right level of abstraction to tackle the stakeholder’s requests, or maybe the right algorithm to predict an important feature didn’t exist yet. At a certain point it seemed that the expectations were immeasurably higher than the available tools. And despite the disillusionment, the projects went on because Process Mining is able to provide valuable insight: the slope of enlightenment can be steep but the efforts are paid back.
From Process Mining to Simulation (and back) with Cardanit
Cardanit is evolving from a pure process and decision editor towards an analysis tool including simulation and optimization features. Applying Process Mining techniques to Cardanit can bring great value to further advance Process Management initiatives.
A first scenario is the dissemination of the results of Process Mining analyses. A web collaborative tool such as Cardanit is the perfect place to show the final process map obtained after manipulating an event log. And the automated report can help the deployment phase of improved processes.
If the event log is rich enough, it is possible to mine not only the process map, but also to infer simulation parameters like processing time for tasks, availability of resources, routing probabilities at gateways and frequency of triggers at start/intermediate events. These analyses can be inserted into the Cardanit simulation interface to create a Digital Twin of your process.
Conversely, the simulation capabilities of Cardanit can provide new data to be analyzed with Process Mining techniques. At the moment you can export only numbers, but soon it will be possible to export also the simulation event log and the comparison with a real one is a valid way of assessing the reliability of the simulation and to measure possible improvements when exploring future scenarios.
Alessandro Turco has a PhD in Applied Math from the International School of Advanced Studies (SISSA) of Trieste and a Master in Management from the School of Management of Milan Polytechnic (MIP). He worked for ESTECO SpA for ten years, starting as a researcher for the Numerical Method Group and then moved to project manager of Cardanit.
Alessandro Turco has a PhD in Applied Math from the International School of Advanced Studies (SISSA) of Trieste and a Master in Management from the School of Management of Milan Polytechnic (MIP). He worked for ESTECO SpA for ten years, starting as a researcher for the Numerical Method Group and then moved to project manager of Cardanit.
A business is only as efficient as its processes. What are you waiting to improve yours?